How Business Intelligence Needs Data Engineering in Finance
- DataEngi
- Feb 18
- 4 min read
In the financial industry, data is everything. From tracking market trends to detecting fraud, companies rely on Business Intelligence (BI) to make informed decisions. BI is only as powerful as the data behind it. That’s where data engineering comes in. Without a solid data infrastructure, financial BI tools cannot provide accurate, timely, and reliable insights.
So, how data engineering powers BI in finance, ensuring high-quality data for risk management, fraud detection, forecasting, and regulatory compliance.
The Role of Data in Financial BI
In finance, data is the foundation of every decision. Whether a company is analyzing investment risks or forecasting revenues, the quality and accuracy of data impact business results. Here are the key aspects of data in financial analytics:
Accuracy and reliability. Since financial decisions are based on accurate data, inaccurate or outdated information can lead to significant economic losses.
Real-time processing. Real-time data streams allow for instant analysis of stock trading, automated risk assessment, and fraud detection.
Data integration. BI systems need to combine data from different sources to provide meaningful insights.
Historical analysis and forecasting. BI tools use historical financial data to identify patterns and predict future trends.
Without data engineering, financial BI systems face incomplete, inconsistent, or inaccessible data, which could lead to poor decision-making.

How Data Engineering Enhances BI in Finance
BI in finance relies on clean, structured data. Data engineering is the foundation that makes this possible. Here's how it's possible:
Creating data pipelines: Data engineers develop ETL/ELT workflows to collect, process, and transform raw financial data into structured formats for business intelligence tools.
Real-time analytics: Data engineers use streaming technologies such as Apache Kafka or Spark to ensure BI systems receive up-to-date financial data.
Data quality assurance: Data engineers set up validation processes to identify errors, remove duplicates, and ensure compliance with financial regulations.
Optimizing data storage and access: They use cloud-based data warehouses (Snowflake, Databricks, BigQuery) to ensure secure and scalable financial data management.
Support for advanced analytics: data engineers prepare and structure data for financial forecasting, risk analysis, and fraud detection based on artificial intelligence.
Financial analytics would be unreliable, slow, and disjointed without data engineering, making accurate decision-making nearly impossible.
Key Use Cases of BI in Finance
So, thanks to data engineering, BI in finance is becoming a powerful tool for making smarter, faster, and safer decisions. Let's take a look at some of the most important use cases:
BI tools analyze transactional data in real time to detect suspicious activity, identify anomalies, and prevent fraud before it happens. Banks use machine learning-based BI dashboards to identify patterns of suspicious transactions, such as unusually large transfers or transfers to known high-risk accounts, in real-time.
Financial institutions must assess and manage risks associated with loans, investments, and regulatory compliance. BI platforms process vast amounts of data to identify potential risks, predict credit defaults, and ensure business compliance with legal standards.
BI allows companies to analyze past financial performance and predict future trends. Companies use BI dashboards to forecast revenue, track expenses, and optimize budget planning based on historical and real-time data.
Financial companies use BI to understand customer behavior, segment customers, and offer personalized financial products (loans, investment plans, insurance).
Investment companies use BI to analyze stock market trends, compare asset performance, and make data-driven investment decisions. Real-time analytics help traders respond to market fluctuations instantly.
BI also helps financial companies analyze internal processes, identify shortcomings, optimize workflows, and cut unnecessary costs.
Key Data Engineering Tools & Technologies for Financial BI
Data engineers use special tools and technologies to ensure that business intelligence in finance works effectively. These solutions help to collect, process, store, and analyze financial data securely and at scale.
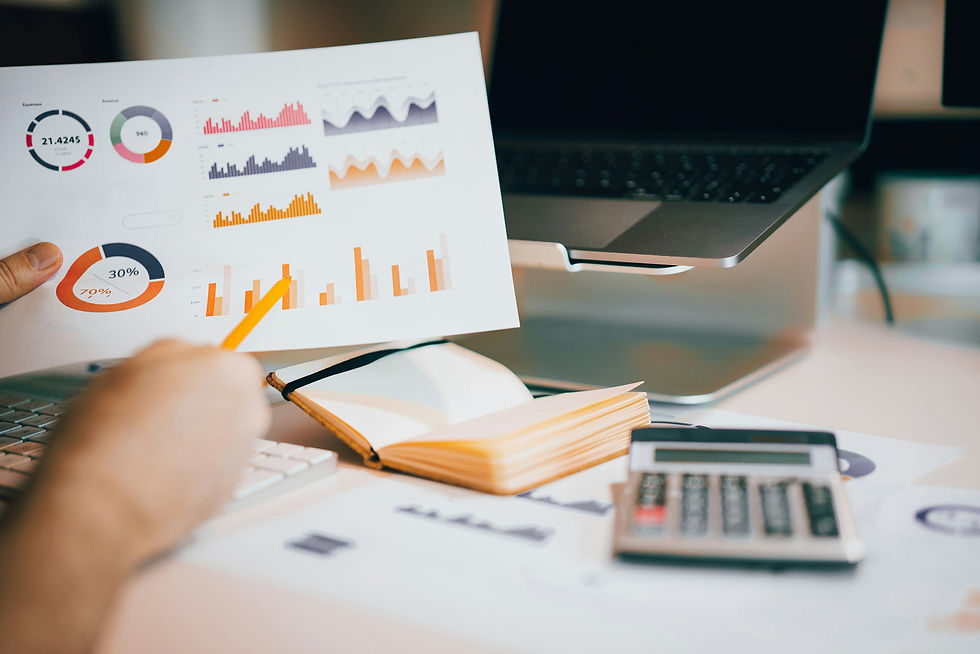
These tools automate the extraction, transformation, and loading of financial data from various sources into BI platforms. They also help data engineers ensure data consistency across banking systems, trading platforms, and regulatory databases.
Data orchestration: Apache Airflow, Dagster, Prefect automate workflows, ensuring uninterrupted and timely processing of financial data. They allow you to plan and control the flow of critical financial data.
Real-time data processing: Apache Kafka, Apache Flink, and Spark Streaming allow you to detect real-time fraud, assess risks, and analyze stock markets.
Instantly process high-speed financial transactions.
Data storage: Snowflake, Google BigQuery, Amazon Redshift, Databricks, Kafka provide storage, efficient management of large amounts of data, and support for quick queries for BI tools and dashboards.
BI and visualization tools: Tableau, Power BI, Looker, Amazon QuickSight, and Metabase allow you to transform structured financial data into interactive dashboards and reports for decision-makers. These tools support data-driven insights into risks and revenue trends.
Financial services companies use these data engineering tools to maintain data integrity, automate workflows, and ensure regulatory compliance, making BI a powerful asset to business success.
So, as we can see, business intelligence is essential to the financial industry, enabling better decision-making, risk management, fraud detection, and forecasting. However, BI is only as strong as the data behind it, and this is where data engineering plays a crucial role.
By creating reliable data pipelines, ensuring real-time data processing, and maintaining security and compliance, data engineers form the foundation for accurate and efficient financial analytics. With the right tools and technologies, financial institutions can fully leverage BI to drive growth, enhance security, and maintain leadership in a competitive marketplace.
Comments